Vegetation Monitoring
Leveraging AI algorithms, we provide comprehensive vegetation monitoring solutions such as accurate tree canopy cover assessments, precise biomass estimation, Urban Heat Island (UHI) estimation, and Normalized Difference Vegetation Index (NDVI). Our platform excels in monitoring vegetation health, detecting temporal changes, monitoring Land Surface Temperature (LST) and aiding in effective conservation strategies. Using advanced AI models, we enable to automatically replace manual work in carbon stock calculations, Land use and land cover prediction to empower environmental initiatives with data-driven insights.
Remote sensing-based AI Solution
Global warming has been a concern since the late nineteenth century. Especially in cities and urban areas, the average land surface temperature (LST) increased more and resulted in urban heat islands (UHIs). Given this fact, we in GeoAI ask further that what in cities lead to the increased LST, and how the linkages between city planning / construction and UHI can be determined.
Traditionally, the difficulty of the analysis is intensive manual labour. Its requiring of human labelling various land use and land cover (LULC) is time consuming and slows down the process, in contrast with the high-speed city construction. With advanced AI technology, we are able to streamline the process without intense labours and use minimal data to achieve comprehensive results. What we need is simply thermal satellite images. Other data such as 360 camera, UAV-LiDAR, and mobile LiDAR is also beneficial to be processed together for better visualization and semantic information.
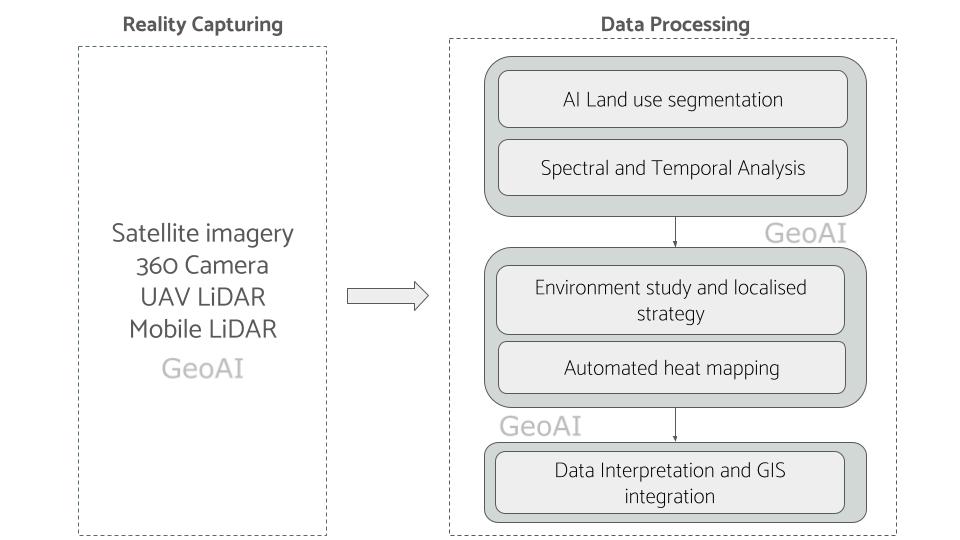
Tree Canopy Modeling
Leveraging the capabilities of a Convolutional Neural Network (CNN), GeoAI excels in delivering finely detailed predictions of canopy cover over expansive areas. This proficiency is honed through the utilization of LiDAR data for vegetation analysis using remote sensing. The accurate data is expected to ensure a comprehensive and accurate understanding of the landscape.
The high-resolution predictions generated by GeoAI serve a dual purpose, extending beyond analysis. They play a crucial role in compliance monitoring and assurance functions, offering an additional layer of high-quality data. This, in turn, supports the verification of geospatial data associated with carbon abatement projects. By harnessing the power of Convolutional Neural network (CNN), GeoAI enhances the reliability and precision of predictions. It contributes to the robustness of monitoring initiatives in the realm of environmental and carbon offset projects.
This innovative approach not only elevates the accuracy of canopy cover and vegetation assessments but also establishes GeoAI as a valuable tool in the broader context of environmental sustainability. The fusion of advanced neural network technology with high-quality data sources positions GeoAI as a key player in ensuring the credibility and effectiveness of initiatives aimed at carbon abatement and geospatial verification.
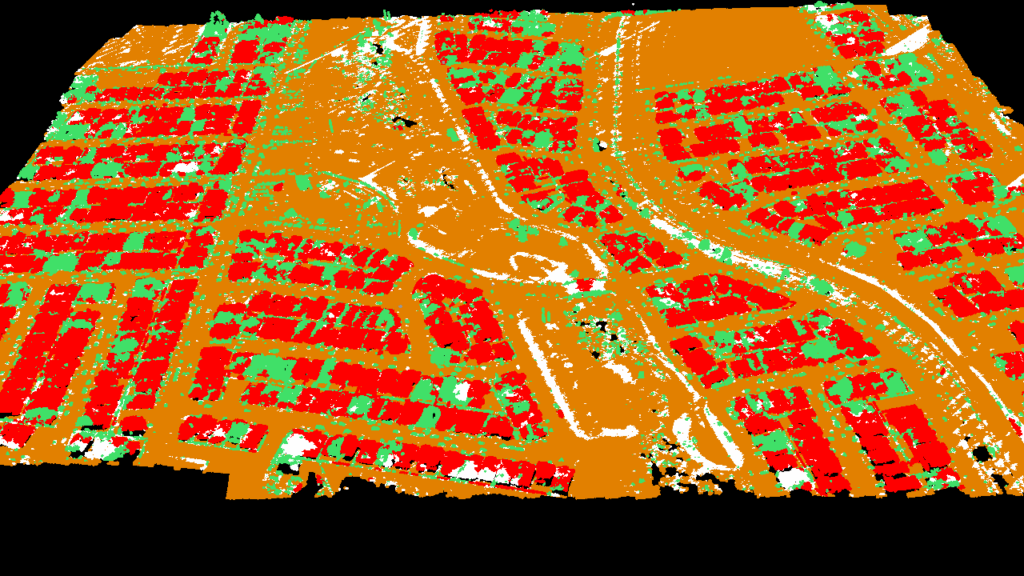
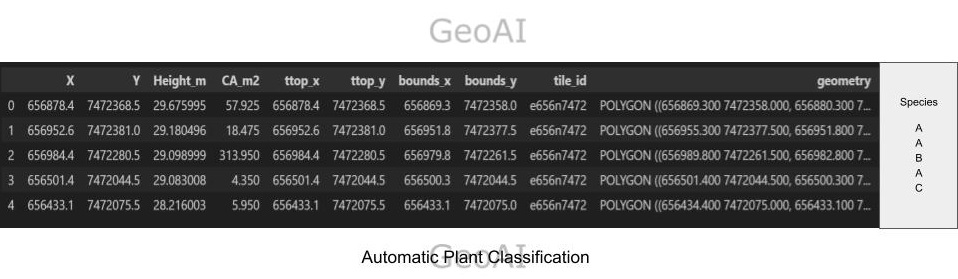
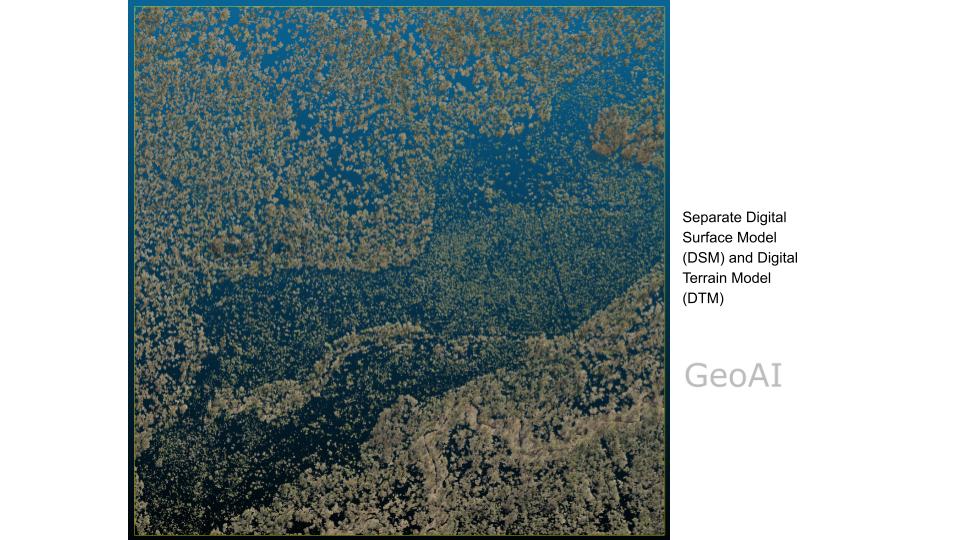
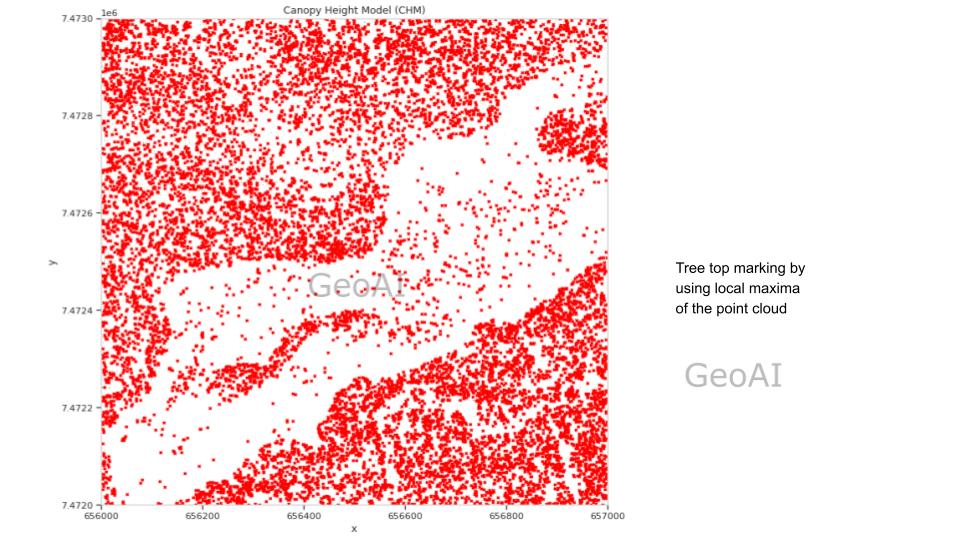
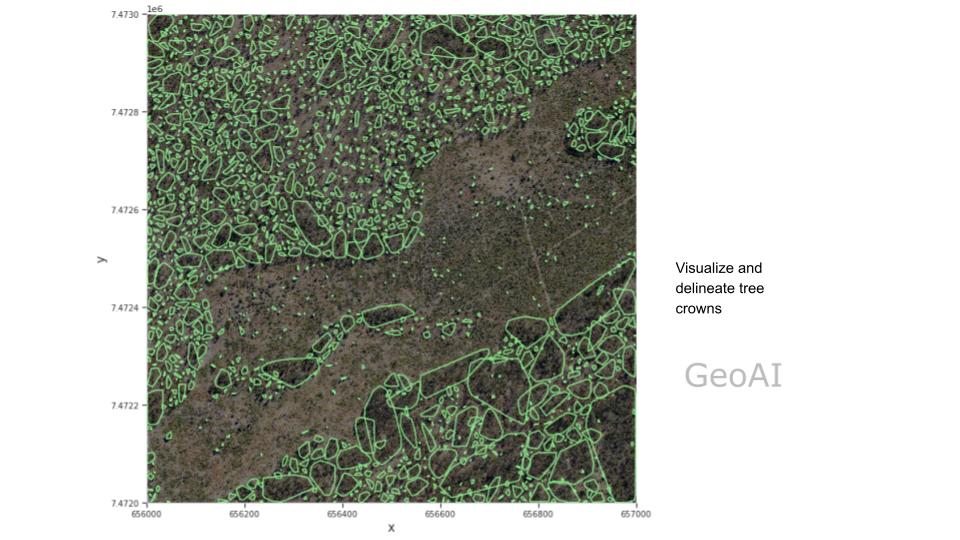
High resolution satellite imagery and LiDAR point cloud data have a great potential to move forest monitoring from field surveys to remote-sensing based AI solution. While there are common methods, they not only require labour intensive feature engineering, but are not able to use spatial and spectral data at the same time.
We propose an AI method that utilise all data at hand, including field notes, spatial data, and spectral data. Deep learning model is trained to extract related features from input and reduce manual work. By examine extracted features from raw data, the model is able to classify tree species without field notes.
Vegetation Mapping and Automatic Plant Classification
Vegetation Mapping and Automatic Plant Classification
We propose an AI-driven method for vegetation mapping and plant classification that leverages all available data, including field notes, spatial information, and spectral data. By employing a deep learning model, the approach extracts meaningful features from raw input data, significantly reducing the need for manual work. The model is capable of analyzing extracted features to accurately classify tree species, even in the absence of field notes. This comprehensive integration of multiple data sources ensures a robust and efficient solution for automating plant classification, paving the way for advanced ecological studies and resource management.
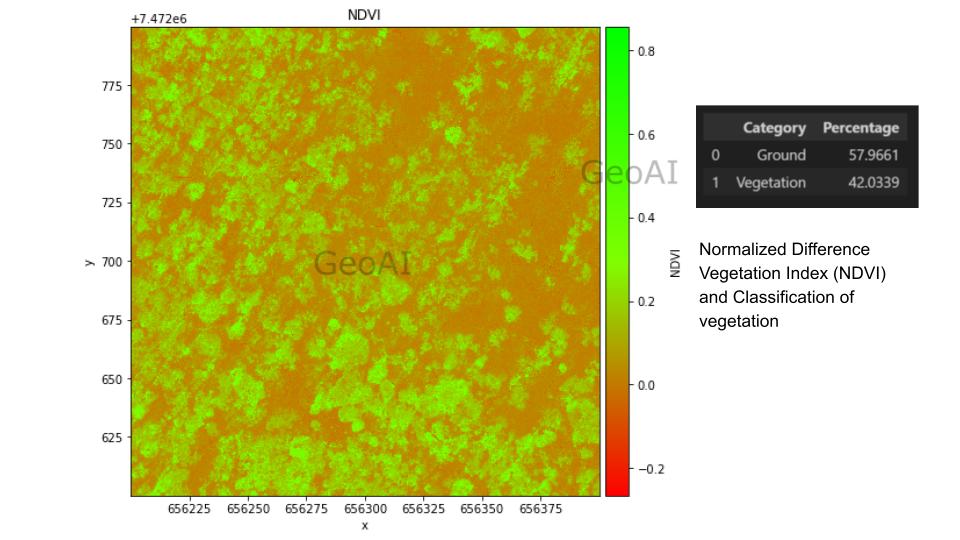
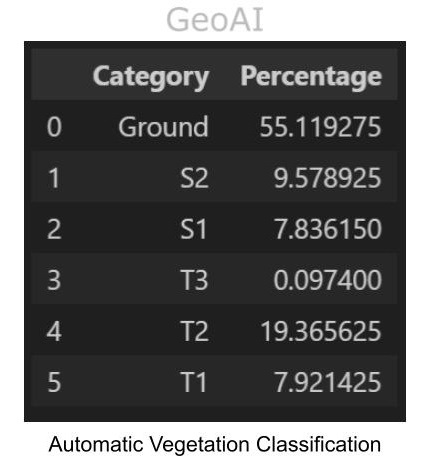
Urban Heat Island (UHI)
The Urban Heat Island (UHI) describes the occurrence of elevated temperatures in urban areas compared to nearby rural regions. This phenomenon is a major environmental issue, largely influenced by urban development and human activities.
GeoAI offers innovative solutions for monitoring and mitigating the Urban Heat Island (UHI) effect. By integrating geospatial data, remote sensing technologies, and advanced AI algorithms, GeoAI can analyze temperature patterns, land use, and vegetation coverage in urban areas. This technology enables the identification of heat hotspots and the assessment of factors contributing to UHI, such as reduced green spaces and high-density infrastructure. Furthermore, GeoAI can support the development of data-driven strategies to combat UHI, such as optimizing urban planning, increasing vegetation coverage, and designing reflective materials for buildings and roads. By providing real-time insights and predictive analytics, GeoAI empowers decision-makers to implement targeted interventions, enhancing urban sustainability and livability.
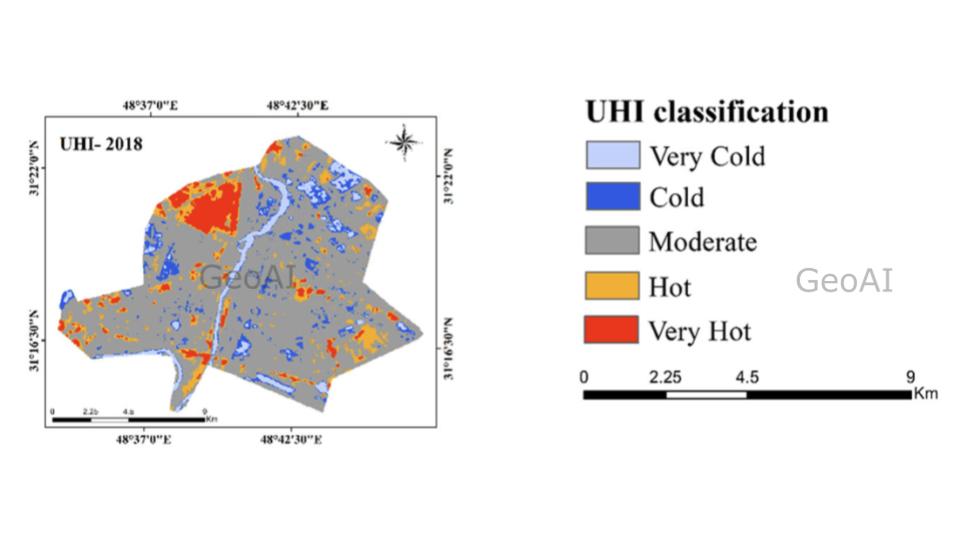
Uncover the secrets of your vegetation analysis with AI-powered insights!
Are you struggling to accurately conducting vegetation assessment? Our cutting-edge platform, powered by AI and remote sensing technology, offers a revolutionary solution for comprehensive vegetation analysis. We delve into the heart of your vegetation ecology, providing data-driven insights that empower you to:
- Gain precise canopy cover assessments to monitor forest health and track changes over time.
- Obtain accurate biomass estimates to inform sustainable management practices.
- Detect and quantify deforestation rates to guide effective conservation strategies.
- Uncover hidden patterns and trends in your vegetation data using advanced data analysis techniques.
- Measuring the Land use and Land Cover utilization (LULC)
- Measuring some indicator such as Normalized Difference Vegetation Index (NDVI), Land Surface Temperature (LST), Urban Heat Island (UHI), Urban Thermal Field Variance Index (UTFVI), Enhanced Vegetation Index (EVI)
Stop relying on outdated methods and embrace the power of AI! Our user-friendly platform makes it easy for anyone to access valuable vegetation monitoring insights, regardless of their technical expertise.