Automatic Plant Classification for Vegetation Mapping
Automatic Plant classification is an important part for vegetation mapping. High-resolution satellite imagery and LiDAR point cloud data are transforming forest monitoring by enabling the shift from traditional field surveys to advanced, remote-sensing-based AI solutions. These technologies hold immense potential for vegetation mapping and plant classification, offering scalable, cost-effective, and accurate insights into forest ecosystems. However, existing methods for plant classification often fall short due to their reliance on labor-intensive feature engineering and their inability to simultaneously utilize both spatial and spectral data effectively.
Challenges with Traditional Methods
- Labor-Intensive Processes: Traditional methods rely heavily on manual feature engineering, requiring significant time and expertise.
- Data Silos: Many methods fail to integrate multiple data types, such as spatial and spectral data, leading to incomplete analysis.
- Limited Scalability: Field surveys are resource-intensive and impractical for large-scale vegetation mapping.
Our AI-Driven Solution
To address these limitations, GeoAI propose an AI-driven method that fully leverages all available data sources, including:
- Field Notes: On-the-ground observations for contextual insights.
- Spatial Data: High-resolution geospatial information derived from LiDAR point clouds.
- Spectral Data: Detailed vegetation characteristics captured through satellite imagery.
Our approach employs a deep learning model trained to extract meaningful features directly from raw input data, significantly reducing the need for manual intervention. By analyzing the extracted features, the model achieves high accuracy in classifying tree species, even when field notes are unavailable.
Key Features of the Proposed Method
- Detect and segment vegetation: By using LiDAR data taken from airborne laser scanner, we can detect and segment vegetation automatically. We train a deep learning model for automatic segmentation of the vegetation area.
- Produce vegetation indexes: By using Tiff spectral data, GeoAI can generate the vegetation indexes. We use a TIff file that contains spectral bands such as near-infrared (NIR) for calculating vegetation indexes. The, we automatically calculate the Normalized Difference Vegetation Index (NDVI) or Enhanced vegetation Index (EVI).
- Match the detected crowns with LiDAR: We match the vegetation segments with the vegetation indexes
- Match past labeled data with LiDAR: By using field notes ground reference, we can match past ground truth data with current information from LiDAR. As a result, we can generate tiles that contains spectral and spacial information.
- Canopy height modeling: The canopy height model (CHM) represents the difference between the top canopy surface and underlying ground topography from LIDAR data. This data can be used to calculate the height of the tree species.
- Combine NDVI and CHM to automatically classify plants into species. A 3D-CNN model needs to be trained. The training data includes all bands and CHM we have gained, as well as the tree crown shape file. In addition, we need to label each row with species as ground truth.
- Automatic Plant Classification: After training, the model is expected to take the same data as input, except species, and output species for each tree ranked on probability.
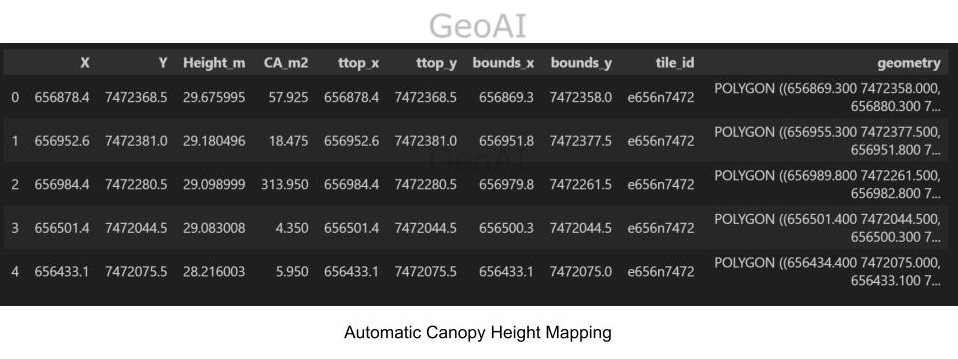
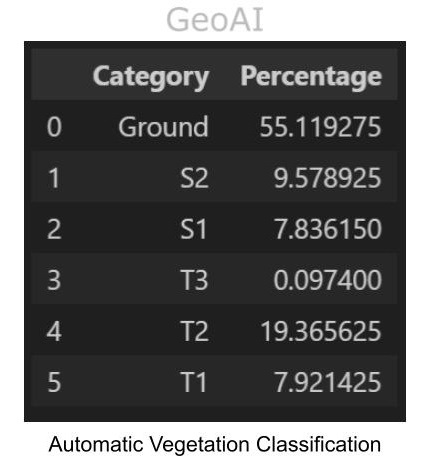
Advantages of AI in Vegetation Mapping
This innovative approach integrates data from multiple sources, enabling the model to capture complex relationships and patterns that are often missed by traditional methods. High-resolution satellite imagery provides detailed spectral data that reveals vegetation characteristics, while LiDAR point cloud data offers precise spatial information about tree structures and canopy heights. Together, these datasets empower the deep learning model to deliver unparalleled accuracy and detail in plant classification.
Some of the key advantages include:
- Efficiency: Reduces dependency on field surveys, saving time and resources.
- Accuracy: Delivers precise classifications by analyzing both spatial and spectral dimensions.
- Scalability: Facilitates large-scale forest monitoring programs.
- Cost-Effectiveness: Minimizes labor costs associated with traditional methods.
Applications of Automatic Plant Classification
The ability to analyze vast landscapes remotely and accurately has wide-ranging applications, such as:
- Biodiversity Conservation: Monitoring and protecting endangered species and habitats.
- Climate Change Mitigation: Assessing carbon storage capacity and forest health.
- Sustainable Forest Management: Informing policies for resource use and restoration efforts.
- Disaster Response: Rapid assessment of forest damage after natural events such as wildfires or storms.
By automating plant classification and vegetation mapping, this solution revolutionizes the way forest ecosystems are studied and managed. With AI-driven insights, researchers and decision-makers can develop data-driven strategies to preserve and restore ecosystems, ensuring a sustainable future for our planet.
Category List
- 3D Point Cloud
- Artificial Intelligence
- Asset Management
- Digital Twin
- Featured
- Hardware
- Knowledge Graph
- LiDAR
- News
- Site Monitoring
- Vegetation Monitoring
- Virtual Reality
Recent Post
- Automatic Plant Classification for Vegetation Mapping
- Vegetation Map Australia
- NDVI Imaging: What It Is and How AI Enhances Its Value
- Understanding the Urban Heat Island Effect: Causes, Impacts, and Solutions
- Land Surface Temperature (LST)