Vegetation Mapping: Revolutionizing Forest Monitoring with High-Resolution Satellite Imagery and LiDAR
Vegetation mapping is an essential tool for understanding and managing forests. It allows researchers and environmental agencies to monitor forest health, biodiversity, and environmental changes. Traditional forest monitoring methods rely heavily on field surveys, which are labor-intensive and often limited in scope. However, with advancements in high-resolution satellite imagery and LiDAR (Light Detection and Ranging) point cloud data, vegetation mapping is transforming into a remote-sensing-based AI solution. By utilizing cutting-edge technology, this method offer a more efficient, comprehensive approach to forest management.
Challenges in Traditional Methods
Conventional vegetation mapping techniques often require extensive feature engineering. It involves manually selecting and designing features that are useful for analyzing vegetation. While effective, these methods are time-consuming, prone to human error, and can’t leverage spatial and spectral data simultaneously. Spatial data provides information on the structure and layout of forests. Meanwhile spectral data captures details like vegetation health and species type. Existing methods struggle to integrate these data types, limiting their ability to accurately map and classify vegetation.
GeoAI Solution for Vegetation Mapping
To address these challenges, GeoAI propose an AI-driven approach that utilizes a combination of high-resolution satellite imagery, LiDAR point cloud data, and even field notes when available. We design this deep learning model to automatically extract and integrate relevant features from spatial and spectral data to reduce the need for manual feature engineering.
By training on a satellite images and colourised Lidar data, the model learns to interpret raw data and capture complex patterns in vegetation structure and health. Unlike traditional methods, this approach allows the model to process spatial and spectral data simultaneously. It provides a more holistic view of forest ecosystems. Importantly, once trained, the AI model can classify tree species with high accuracy, even without additional field notes. This approach make it a powerful tool for remote forest monitoring.
Advantages of AI in Vegetation Mapping
- Automated Feature Extraction: The AI model automates the process of feature extraction, and classification. It is aimed to minimize the need for manual intervention and reducing labor costs. This automation is particularly valuable when dealing with large-scale data from satellites and LiDAR.
- Integration of Multi-Source Data: By combining spatial and spectral data, the model provides a more comprehensive understanding of forest characteristics and segmentation. By using this method, we aim to improve the accuracy in vegetation mapping. This integration allows for better differentiation between ground, trees, and species.
- Enhanced Tree Species Classification: The AI model can classify tree species by analyzing patterns in spectral and spatial data. These data are correlated with unique characteristics of different species. This capability is a game-changer, as it enables precise vegetation mapping without requiring field notes, making it suitable for remote and hard-to-access regions.
- Scalability and Efficiency: Traditional field surveys are limited by time and accessibility. However, AI-based approach allows for rapid, large-scale analysis. High-resolution satellite imagery and LiDAR data cover vast forested areas, providing continuous monitoring over time.
The Role of Deep Learning in Vegetation Mapping
Deep learning models excel at handling large, complex datasets and can learn intricate patterns within spatial and spectral data. The model in our approach uses convolutional neural networks (CNNs) to identify specific vegetation features, such as tree structure, canopy density, and spectral signatures unique to different tree species. Through extensive training, the model becomes capable of recognizing these features autonomously, enabling it to perform vegetation mapping at a level of accuracy that rivals or even surpasses traditional methods.
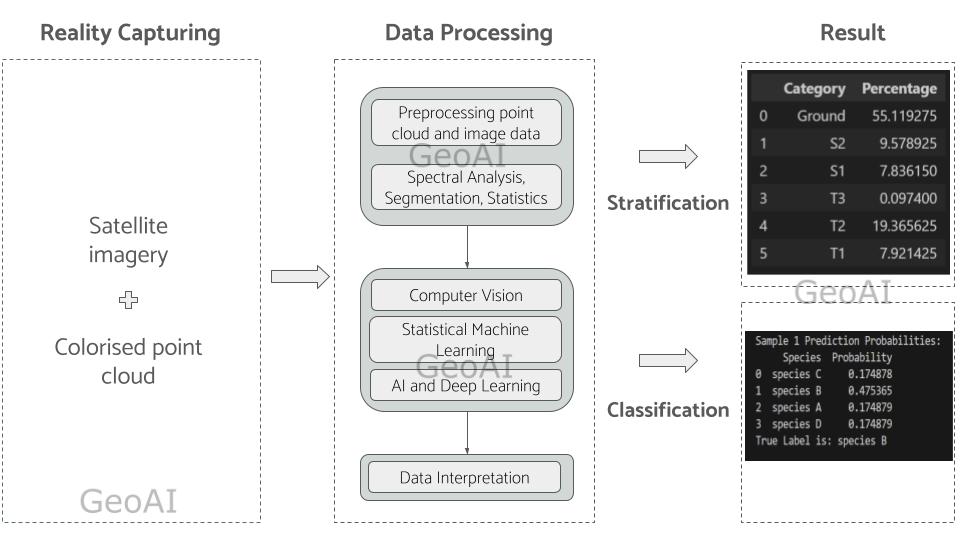
The integration of high-resolution satellite imagery and LiDAR data with AI presents a transformative approach to vegetation mapping and forest monitoring. By leveraging spatial and spectral data, this AI-based solution provides a detailed, accurate view of forest ecosystems while reducing the need for field surveys. As remote-sensing technologies continue to improve, AI-driven vegetation mapping will play an increasingly vital role in sustainable forest management, biodiversity conservation, and climate change mitigation.
Category List
- 3D Point Cloud
- Artificial Intelligence
- Asset Management
- Digital Twin
- Featured
- Hardware
- Knowledge Graph
- LiDAR
- News
- Site Monitoring
- Vegetation Monitoring
- Virtual Reality
Recent Post
- Photogrammetry vs Image Processing: What’s the Difference and How Are They Used?
- Soil Adjusted Vegetation Index (SAVI): Definition and Application
- NDWI (Normalized Difference Water Index): Identifying Water Bodies with Remote Sensing
- Construction Management in the Digital Era: How GeoAI Transforms Projects with Data Analytics and AI
- What is Near Infrared (NIR)?