3D Point Cloud: Tools, Processing and Application
What is 3D Point Cloud?
Point Cloud is Three Dimensional (3D) data represent physical object in 3D coordinate system. Think of a point cloud as a million tiny dots. Each dot represents a single measurement, capturing the precise location of a specific point in space with an x, y, and z coordinate. By gathering billions of these points, we can build an accurate 3D representation any object or environment virtually.
Unlike traditional 2D images, which can only capture surfaces, 3D point clouds can preserve depth and dimension. Therefore, by generating 3D point cloud data, we will not only reveals the facade, but also the inner side of an object. Having this capability, the application of 3D point cloud is ranging from medical imaging, manufacturing, construction, 3D gaming, 3D printing, and Virtual Reality (VR).
Generation of Point Cloud Data
3D point cloud data, with its intricate point-based reconstructions of the world, has revolutionized how we capture and analyze our surroundings. But how do we actually generate these data? Point cloud data can be generated by using different methods such as laser scanners, LiDAR sensors, or even specialized cameras. Let’s dive into the fascinating world of point cloud generation methods.
1. Laser Scanning
A laser beam rapidly sweeps across a target object or environment, sending back precise distance measurements for each point it hits. These measurements are then translated into 3D coordinates, forming the foundation of the point cloud.
2. LiDAR (Light Detection and Ranging)
Mounted on planes, drones, or even cars, LiDAR systems emit laser pulses and record the reflected light’s travel time to generate accurate 3D maps of vast areas. This makes it ideal for surveying landscapes, forests, and even entire cities.
3. Structured Light Scanning
This method projects a grid of light patterns onto an object. Cameras capture the distortions in the projected pattern as it interacts with the object’s surface, allowing software to calculate the precise 3D coordinates of each point. This technique is often used for capturing smaller, intricate objects with high detail.
4. Photogrammetry
By analyzing overlapping photographs of an object from different angles, specialized software can reconstruct its 3D shape. This method is less expensive than the others but often results in less accurate point clouds, particularly for complex objects or scenes with poor lighting.
5. Time-of-Flight (ToF) Cameras:
These specialized cameras measure the time it takes for emitted light pulses to bounce back from an object, directly calculating the distance to each point. This allows for real-time 3D point cloud generation, making it ideal for applications like robotics and augmented reality.
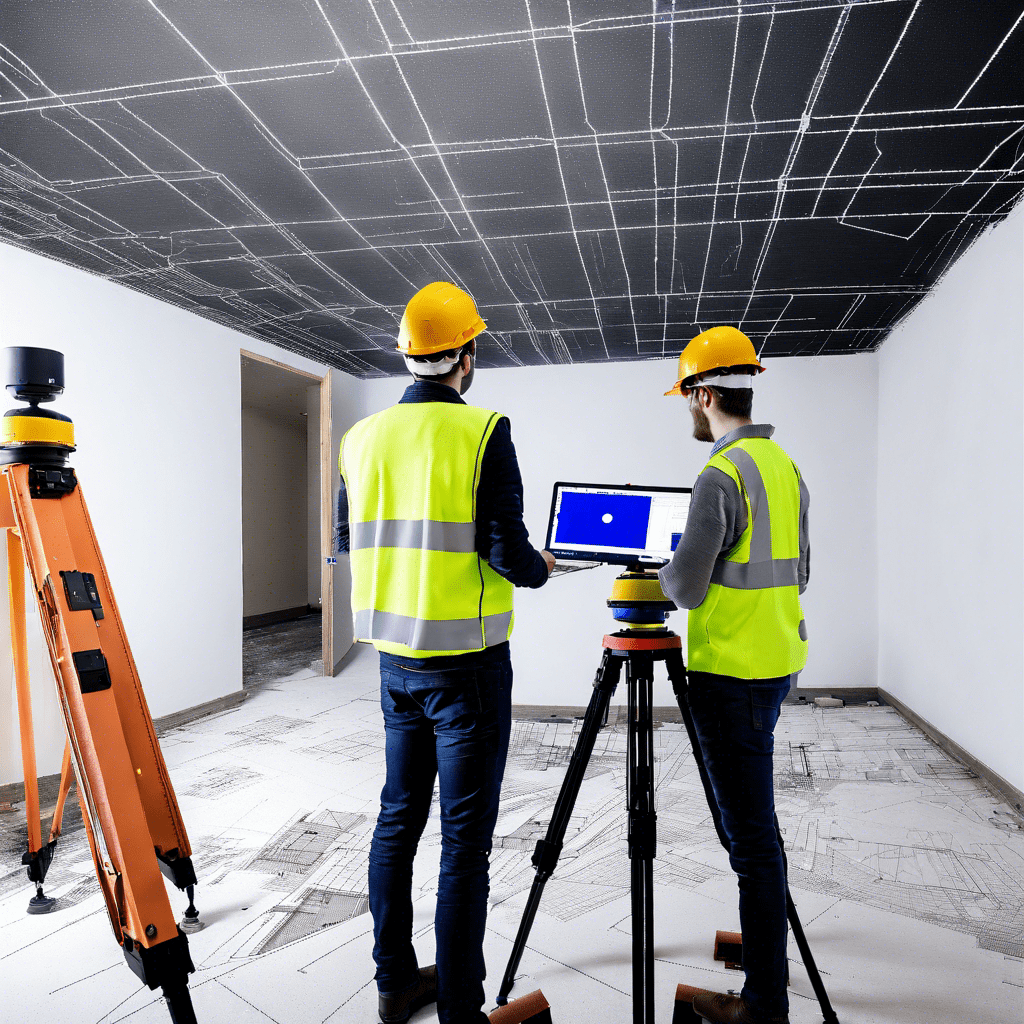
These are just some of the main methods for generating 3D point cloud data. As technology advances, hybrid approaches combining different techniques are becoming increasingly common, leading to even more accurate and detailed point clouds. Remember, the choice of method depends on factors like the size and complexity of the object, desired level of accuracy, and budget constraints.
What information Stored in 3D Point Cloud Data?
Each point in the point cloud represents a specific coordinate in 3D space and may contain additional information depending on how it was captured. We can find some common types of data in a 3D point cloud as below:
- Geometric Coordinates: Each point in the point cloud is defined by its spatial coordinates in three-dimensional space (x, y, z), representing its position relative to a reference frame.
- Color or Intensity: Point clouds generated from photographs or laser scans may include information about the color or intensity of each point. This color data can be used to add visual realism to 3D models or provide additional context for analysis.
- Reflectance or Intensity Values: In LiDAR point clouds, each point may be associated with a reflectance value or intensity value, which indicates the amount of light reflected or returned from the surface at that point. This information can be useful for distinguishing between different materials or surfaces.
- Classification or Segmentation: Point clouds can be classified or segmented based on characteristics such as object type, surface type, or vegetation density. This classification enables the extraction of specific features or objects from the point cloud and supports various applications such as urban planning, forestry management, or object detection.
- Normal Vectors: Normal vectors represent the orientation or direction of each point’s surface. They provide information about the local geometry of the surface. We are commonly use the normal vector for tasks such as surface reconstruction, mesh generation, or lighting simulations.
- Time Stamp or Acquisition Metadata: Point clouds may include metadata such as the time stamp of data acquisition, sensor parameters, or GPS coordinates. This information helps in data alignment, registration, and fusion when combining multiple point clouds captured at different times or from different sensors.
Overall, 3D point clouds contain a wealth of information about the geometry, appearance, and characteristics of the captured scene, making them valuable assets for a wide range of applications in fields such as surveying, mapping, urban planning, archaeology, robotics, and more.
3D Point Cloud Data Processing
While a 3D point cloud captures the intricate geometry of a scene, it does not have semantic information regarding the object. Each point cloud could contain information such as 3D coordinate, intensity, and colour (RGB) based on the tools to generate the data. To unlock its secrets and extract relevant information, we need to carefully process this data.
The process including pre-processing to remove noise, segmentation to grouping dots into clusters, feature extraction to get key characteristic of each segmented object, classification to assign semantic meaning to the object, and visualization. In order to process the point cloud data, there are different software commonly used to process the point cloud data. For general purpose, there is an opensource software such as CloudCompare and Meshlab. Meanwhile, some software are designed for a specific industry. In architecture, engineering, and construction there is Autodesk ReCap and Trimble RealWorks. For surveying and mapping there is Leica Cyclone, Terrasolid, and Pix4D.
Application of 3D Point Cloud
3D point clouds are revolutionizing how we capture, analyze, and interact with the world around us. By capturing millions of precise points with specialized sensors, we can create detailed 3D representations of any object. But the magic doesn’t stop there; these point clouds unlock a treasure trove of applications across diverse industries:
1. Medical Imaging
Imaging has gone beyond the slice! 3D point clouds from CT scans allow surgeons to visualize complex bone structures, tumors, and blood vessels with unparalleled precision. This leads to better diagnosis, improved surgical planning, and personalized treatment options.
2. Manufacturing
Precision meets efficiency. Point clouds can map intricate production lines, track object movement in real-time, and identify defects with laser focus. This optimizes quality control, streamlines production processes, and reduces downtime.
3. Construction
From blueprint to reality, point clouds bridge the gap. By scanning existing structures or monitoring construction progress, architects and engineers gain precise measurements, identify potential issues, and ensure project accuracy. Point cloud to 3D model is one of the common method to develop an as-built model of a built environment.
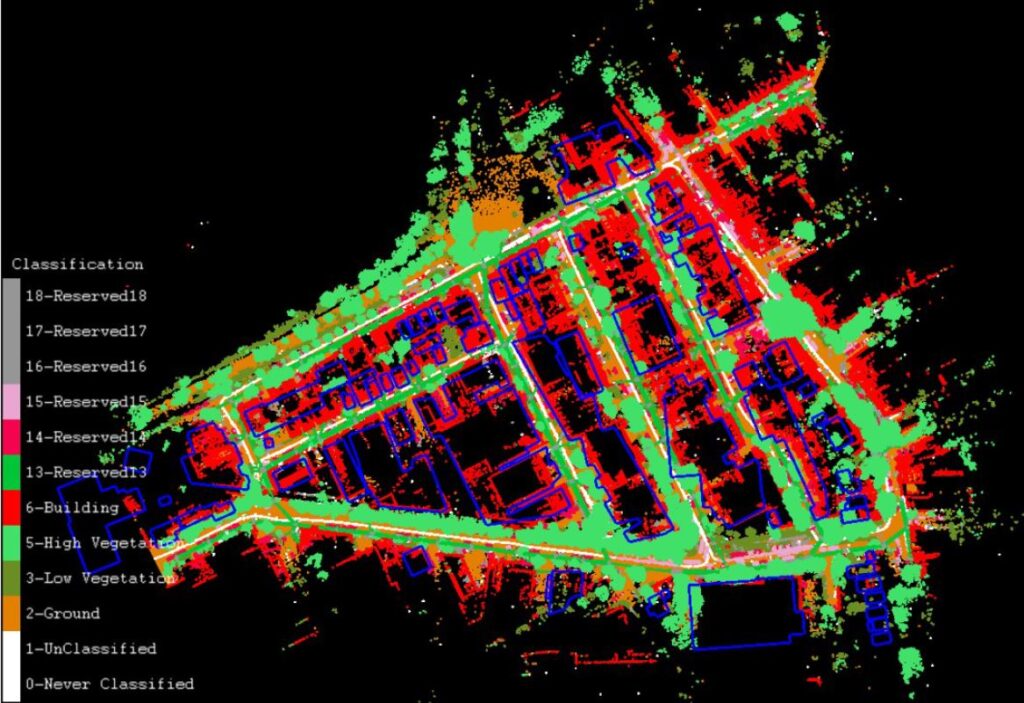
4. 3D Gaming
Immerse yourself in breathtaking worlds. Gaming industry utilize 3D point cloud scan to build a foundation for realistic game environment. It can capture the minute details of landscapes, buildings, and even foliage.
5. 3D Printing
From digital dreams to tangible objects. We can directly convert Point cloud data into 3D printable models. It will open limitless possibilities for prototyping, product customization, and even on-demand manufacturing.
6. Virtual Reality (VR)
Step into the point cloud! By translating point cloud data into VR experiences, users can explore environments, interact with objects, and gain a deeper understanding of spatial relationships in a way never before possible.
These are just a few examples; the possibilities are endless. Point cloud data can transform and enrich countless industry from cultural heritage preservation to autonomous vehicle. And as technology advances, expect even more exciting applications to emerge. Finally, this technology is not just changing how we see the world, it’s changing how we interact with it, one tiny point at a time.
Category List
- Artificial Intelligence
- Asset Management
- Digital Twin
- Featured
- Hardware
- Knowledge Graph
- LiDAR
- News
- Site Monitoring
- Vegetation Monitoring
- Virtual Reality
Recent Post